Nvidia Pushes Deep Learning into Datacenters, IoT
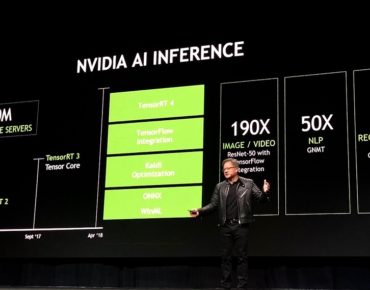
Nvidia upped the ante on GPU acceleration for deep learning with the latest release of inferencing engine that supports a range of machine intelligence applications running in datacenters as well as emerging automotive and robotics applications.
Along with a new version of its TensorRT inference software integrated with Google’s TensorFlow framework, Nvidia (NASDAQ: NVDA) also announced a partnership with chip specialist Arm to integrate deep learning inference into Internet of Things (IoT) and other mobile devices. Both initiatives were announced this week at a company event in San Jose., Calif.
In addition to tighter integration with TensorFlow, Nvidia’s flood of announcements also includes GPU optimization of the popular Kaldi speech recognition framework as well as machine learning partnerships with Amazon (NASDAQ: AMZN), Facebook (NASDAQ: FB) and Microsoft (NASDAQ: MSFT).
The upshot, the graphics chip leaders asserts, are substantial gains in GPU inference compared with CPUs as more datacenter operators seek to deliver deep learning-based services. TensorRT 4 “takes a trained neural network and processes it, compiles it down to a target platform to run in real-time in production use cases,” said Ian Buck, general manager of Nvidia’s Accelerated Computing unit.
As hyper-scale datacenter operators hustle to handle more deep-learning inference tied to “intelligent applications” and frameworks, Nvidia is touting GPU acceleration as a way to achieve real-time performance for the underlying neural networks. “We can now improve the quality of deep learning and help reduce the cost for 30 million hyper-scale servers,” Buck claimed.
The company said Tuesday (March 27) its TensorRT 4 software speeds deep learning inference across a range of enterprise applications by deploying trained neural networks in datacenters as well as automotive and embedded IoT platforms running on GPUs. The company claims up to 190 times faster deep learning inference for applications such as computer vision, machine translation, automatic speech recognition and synthesis as well as recommendation systems.
Nvidia said TensorRT 4 has been integrated into TensorFlow 1.7 to make it easier for developers to run deep learning inference applications on GPUs. The result is higher inference throughput on AI platforms based on Nvidia’s Volta Tensor Core platform. The combination would boost performance for GPU inference running on TensorFlow, according to Rajat Monga, Google’s engineering director.
Along with an AI partnership with Microsoft for Windows 10 applications, Nvidia also announced GPU acceleration for the Kubernetes cluster orchestrator. The goal is to ease deployment of deep learning inference within multi-cloud GPU clusters. Nvidia said it is also contributing GPU enhancements to the Kubernetes developer community.
The datacenter thrust seeks to convince hyper-scale operators to replace racks of mostly x86-based servers with GPU accelerators as they struggle to keep pace with more deep learning inference applications and services. Among other advantages, Nvidia pitches its Tesla-based servers as a way to open up rack space in datacenters while boosting performance and reducing energy costs.
“We have been advancing our platform for HPC and AI dramatically in the datacenter by thinking about the entire stack,” noted Nvidia’s Buck.
A similar thrust into the embedded and self-driving car markets is tied to training deep neural networks on Nvidia’s DGX platform running in datacenters. Those models would then be deployed in embedded devices and autonomous vehicles, for example. The goal is “real-time inferencing at the edge” of the network, the company said.
Another Nvidia partner, chip intellectual property vendor Arm, is heavily focused on IoT processing applications. Last month, Arm introduced Project Trillium, an effort to bring machine learning and neural networking functionality to edge devices via a suite of scalable processors. Project Trillium also includes links to neural networking frameworks such as Google’s (NASDAQ: GOOGL) TensorFlow and the Caffe deep learning apps.
Nvidia and Arm announced a partnership this week to combine Nvidia’s deep learning accelerator framework with Arm’s machine learning platform. The goal is to help IoT chip makers integrate deep learning into their designs as inferencing emerges as a key capability for future IoT devices, noted Rene Haas, president of Arm’s IP Group.
Nvidia’s deep learning accelerator is based on its “autonomous machine system-on-chip” dubbed Xavier. The integration with Arm’s Project Trillium would give deep learning developers another way of adding inferencing into IoT, mobile and embedded chip designs, the partners said.
--Tiffany Trader in San Jose contributed to the report.
Related
George Leopold has written about science and technology for more than 30 years, focusing on electronics and aerospace technology. He previously served as executive editor of Electronic Engineering Times. Leopold is the author of "Calculated Risk: The Supersonic Life and Times of Gus Grissom" (Purdue University Press, 2016).